When Grains Go Rogue: Rethinking Microstructure Growth in Materials Science
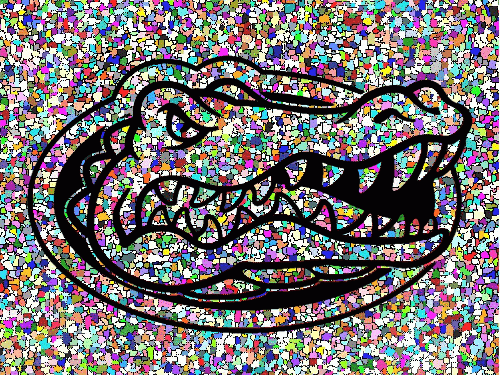
Disclaimer: this is an AI-generated article intended to highlight interesting concepts / methods / tools used within the SmartDATA Lab’s research. This is for educating lab members as well as general readers interested in the lab. The article may contain errors.
New experiments challenge decades-old models of grain growth, revealing a more chaotic—and fascinating—reality.
In the world of materials science, grain growth has long been considered a well-understood process. The prevailing theory posits that, over time, the tiny crystalline grains within a metal or ceramic coalesce and grow, driven by the reduction of total grain boundary area—a process akin to soap bubbles merging to minimize surface tension. This phenomenon, often described by the mean curvature flow model, suggests a smooth, predictable evolution of microstructures.
However, recent experimental observations have begun to unravel this tidy narrative. Instead of the uniform growth predicted by classical models, researchers are witnessing abnormal grain growth (AGG)—a scenario where certain grains grow disproportionately large, consuming their neighbors and leading to a bimodal grain size distribution. This unexpected behavior has significant implications for the mechanical properties of materials, potentially compromising their strength and durability.
The Classical Model: A Brief Overview
Traditionally, grain growth has been modeled using the concept of mean curvature flow, where the velocity of a grain boundary is proportional to its curvature. Mathematically, this relationship is expressed as:
v=Mγκv = M \gamma \kappav=Mγκ
Here, vvv represents the grain boundary velocity, MMM is the mobility, γ\gammaγ is the grain boundary energy, and κ\kappaκ is the curvature. This equation implies that regions with higher curvature (i.e., smaller grains) will shrink, while flatter regions (i.e., larger grains) will grow, leading to a uniform grain size over time.
This model has been the cornerstone of our understanding of grain growth for decades, providing a simplified yet effective framework for predicting microstructural evolution.
The Emergence of Abnormal Grain Growth
Despite the success of the classical model, numerous experiments have reported deviations from its predictions. In particular, the phenomenon of abnormal grain growth—where a few grains grow much larger than the rest—has been observed in various materials, including aluminum alloys and thin films. These observations suggest that factors beyond curvature-driven growth are at play.
Recent studies have identified several potential contributors to AGG:
- Grain Boundary Anisotropy: Variations in grain boundary energy and mobility can lead to preferential growth of certain grains.
- Impurities and Second-Phase Particles: The presence of impurities or particles can pin grain boundaries, inhibiting growth in some areas while allowing others to expand freely. Nature
- Complexion Transitions: Changes in the structure of grain boundaries (known as complexions) can alter their mobility, leading to uneven growth. Nature
These factors introduce a level of complexity that the classical model fails to capture, necessitating a reevaluation of our theoretical frameworks.
Mathematical Modeling: Beyond Mean Curvature Flow
To account for the complexities of AGG, researchers are developing more sophisticated mathematical models. One approach involves incorporating grain boundary anisotropy into simulations, allowing for variations in energy and mobility across different boundaries. This leads to a more accurate representation of microstructural evolution. Physical Review
Another promising avenue is the use of graph-based models, where grains are represented as nodes in a network, and their interactions are governed by edge weights corresponding to boundary properties. This framework leverages concepts from linear algebra, particularly eigenvalue analysis, to predict the stability and growth tendencies of individual grains.
These advanced models offer a more nuanced understanding of grain growth, capturing the stochastic and heterogeneous nature of real-world materials.
Implications for Material Design and Engineering
The recognition of AGG and its underlying mechanisms has significant implications for material design and processing. Engineers must now consider the potential for abnormal growth when developing materials for critical applications, such as aerospace components or microelectronics, where uniform grain structures are essential for performance and reliability.
To mitigate AGG, strategies such as grain boundary engineering, controlled impurity addition, and thermal treatment optimization are being explored. These approaches aim to stabilize grain structures and suppress the conditions that lead to abnormal growth.
Conclusion: Embracing Complexity in Materials Science
The study of grain growth is undergoing a paradigm shift, moving away from simplistic models toward a more comprehensive understanding that embraces the inherent complexity of materials. By integrating experimental observations with advanced mathematical modeling, researchers are uncovering the rich tapestry of factors that govern microstructural evolution.
As we continue to refine our theories and tools, we inch closer to the goal of designing materials with tailored properties, optimized for their intended applications. In this endeavor, acknowledging and embracing the deviations from classical models is not a setback but a necessary step forward.
Relevant Lab Papers
- Yan, W., Melville, J., Yadav, V., Everett, K., Yang, L., Kesler, M. S., Krause, A. R., Tonks, M. R., & Harley, J. B. (2022). A Novel Physics-Regularized Interpretable Machine Learning Model for Grain Growth. arXiv preprint arXiv:2203.03735. Link
- Melville, J. F., Yadav, V., Yang, L., Krause, A., Tonks, M., & Harley, J. B. (2023). A New Efficient Grain Growth Model Using a Random Gaussian-Sampled Mode Filter. SSRN. Link
- Harley, J. B. (2019). Harley Uses Deep Learning to Understand Abnormal Grain Growth. University of Florida ECE News. Link
Other Relevant Literature
- Qiu, C., Srolovitz, D. J., Rohrer, G. S., Han, J., & Salvalaglio, M. (2024). Why is Grain Growth Not Curvature Flow? arXiv preprint arXiv:2411.15983. Link
- Naghibzadeh, S. K., Xu, Z., Kinderlehrer, D., Suter, R., Dayal, K., & Rohrer, G. S. (2024). Impact of Grain Boundary Energy Anisotropy on Grain Growth. Physical Review Materials, 8(9), 093403. Link
- Krill, C. E., Holm, E. A., Dake, J. M., Cohn, R., Holíková, K., & Andorfer, F. (2023). Extreme Abnormal Grain Growth: Connecting Mechanisms to Microstructural Outcomes. Annual Review of Materials Research, 53, 319-345. Link
- Yan, W., Melville, J., Yadav, V., Everett, K., Yang, L., Kesler, M. S., Krause, A. R., Tonks, M. R., & Harley, J. B. (2022). A Novel Physics-Regularized Interpretable Machine Learning Model for Grain Growth. arXiv preprint arXiv:2203.03735. Link
- Melville, J. F., Yadav, V., Yang, L., Krause, A., Tonks, M., & Harley, J. B. (2023). A New Efficient Grain Growth Model Using a Random Gaussian-Sampled Mode Filter. SSRN. Link